Highlights:
Precision medicine promises improved health outcomes but requires more data, diagnostics, and long-term monitoring than current healthcare standards.
New tools utilizing AI are able to advance personalized medicine by making it easier and more accurate to interpret the vast troves of data needed for personalization.
Oncology and BioPharma are two sectors where AI for precision care are the furthest along the technology adoption curve.
Oncology because of the significant variety of cancer types and the evolving ideas on how personal factors affect the disease progression and treatment options.
BioPharma because it is an area where AI is already realizing cost savings by improving clinical trial design and understanding of research outcomes.
Innovators who are pioneering applications of AI cite that access to quality clinical data is one of the top challenges to training models and achieving highly accurate AI enabled tools. In the US, clinical data is fragmented and hard to access.
Several advancements in AI technology are reducing the cost and effort required to build new AI based solutions. Notably: pre-trained models, improvements in hardware and partnership programs such as the Mayo Clinic's Platform Accelerate that grant startups access to data for training models.
Table of Contents
How Personalized Medicine is Different from Traditional Medicine
How the Patient Journey Changes with Precision Medicine
Personalized Medicine in Healthcare Today
AI is Critical to Analyzing Big Data
Does Machine Learning Improve Diagnostic Accuracy?
Advancements in AI Driving Personalized Medicine Forward
Better Access to Data For Rapid Product Development
Hardware and Algorithm Research Reducing Cost
Open Access and Pre-Trained Models Simplifying Product Development
Doctors' and Patients' Adoption of New Technologies
Pain Points in Integrating AI into the Healthcare System
Cost Constraints in Commercializing Precision Medicine
Pharmaceutical Industry: The First to Adopt AIs
Crossing the Chasm to Widespread Adoption
Case Study: Cellular Vehicles – Automating the Last Mile of Cell Therapy to Enable Precision Medicine
Future Applications of AI in Healthcare
Clinical Decision Making
Improving Access and Reducing Cost
About the Applications of AI in Healthcare Research Event
About the Research
Methods of Research
How Personalized Medicine is Different from Traditional Medicine
Personalized medicine shifts from one-size-fits-all treatments to tailored care, using individual data like genetics and lifestyle to customize treatment plans. With personalized medicine, doctors do not use the same treatment for everyone. Instead, they create plans that fit each individual. This can lead to better diagnoses, tailored treatment plans, and better health overall.
"Arriving at care that is truly personalized but also scientifically validated requires a data driven systems approach to wellness. It takes medicine that is predictive and preventive - which, as we will see, is only possible with a vast array of measurements, assessing many of the hundreds of biological networks functioning within the body - to identify what wellness is uniquely for each person."
(Hood and Price, 2023)
For precision medicine to become widespread and effective for most patients, new technologies and techniques are needed to deal with the vast amounts of data and models for both prediction and personalization. As noted by UC Davis, precision medicine intersects with domains to tailor care specifically to the needs of individuals and populations, empowering patients to take charge of their own wellness. (UC Davis Health Center for Precision Medicine and Data Sciences, n.d.)
Inherently, precision medicine is a challenge of data collection, data processing, analysis, and interpretation. Because of this application of AI technology to personalized medicine is particularly promising.
How the Patient Journey Changes with Precision Medicine
In personalized (or precision) medicine, the patient journey shifts from a generalized approach to one that is highly tailored to the individual. Rather than diagnosing and treating based on population-wide data, doctors consider a patient's genetic makeup, lifestyle, and environment from the start. Advanced tools like genomic sequencing and biomarker analysis are used to deliver more accurate diagnoses, while treatment plans are specifically designed to address the patient's unique characteristics. Continuous monitoring with real-time data allows for earlier interventions - even before patients become symptomatic.
In contrast, traditional treatments rely on standardized protocols and generalized data. Diagnosis is often symptom-driven, and treatments follow established guidelines that work for the majority of patients. Adjustments to treatments are typically reactive, made only when a patient shows a lack of response or experiences side effects.
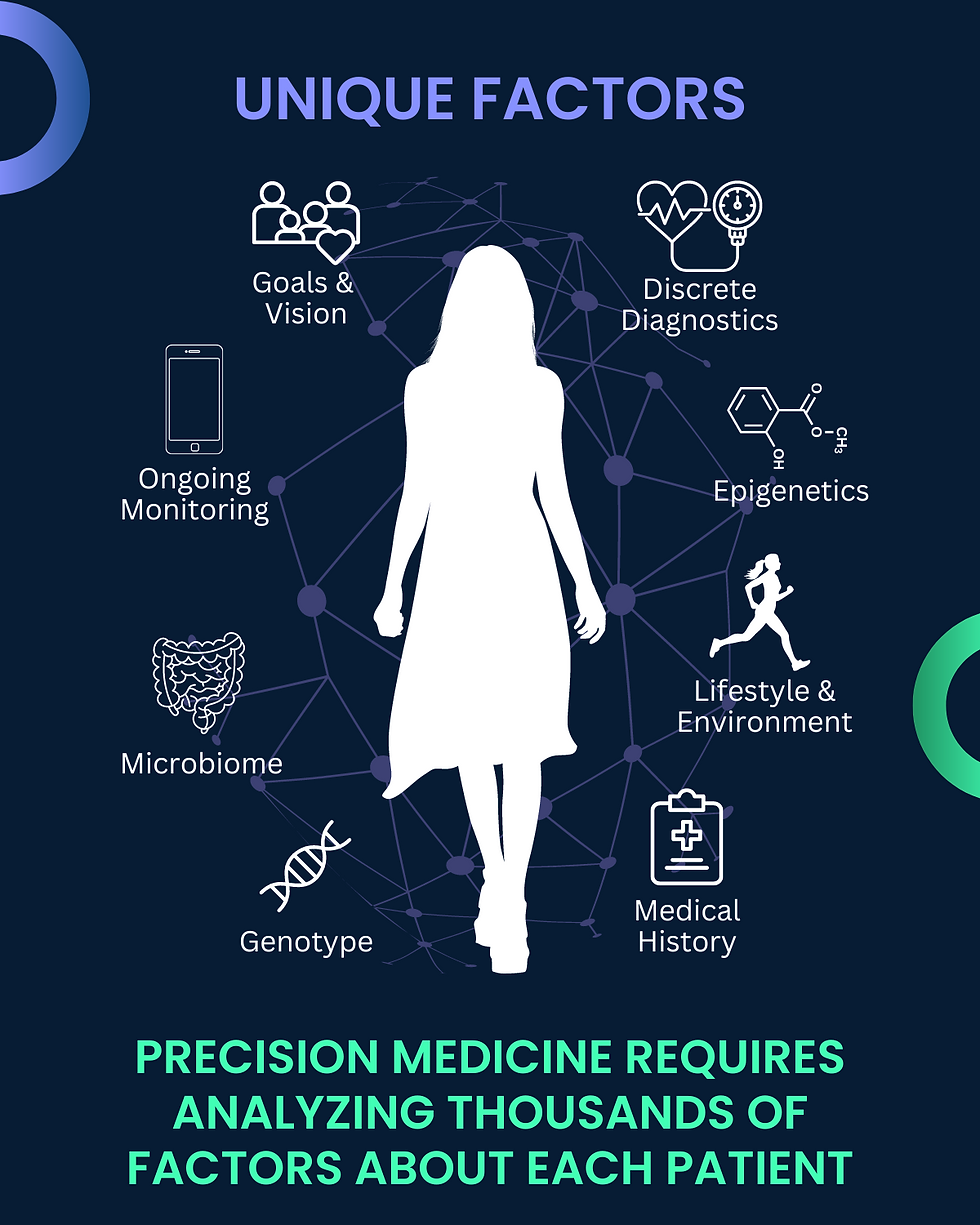
In The Age of Scientific Wellness, authors Hood and Price (2023) write that one recent experiment on individual microbiomes showed that the microbes present in our gut altered the chemical structures of various pharmaceuticals. Up to 13% of drugs tested were altered by our bodies. "Intriguingly, which drugs were altered in this way differed from person to person." Moreover, we now know that microbiomes of people become more and more individualized over time and vary even between family members and members of the same household. A personalized medicine approaches focus on first learning about the factors that are different for each patient and then carefully monitoring effects of interventions, fully recognizing that individual responses vary due to many innate and even changing factors.
Personalized Medicine in Healthcare Today
Personalized medicine is growing, especially in oncology, where treatments are tailored to individual genetic mutations. Targeted therapies, such as those developed based on research from The Cancer Genome Atlas (TCGA), are now part of standard care.
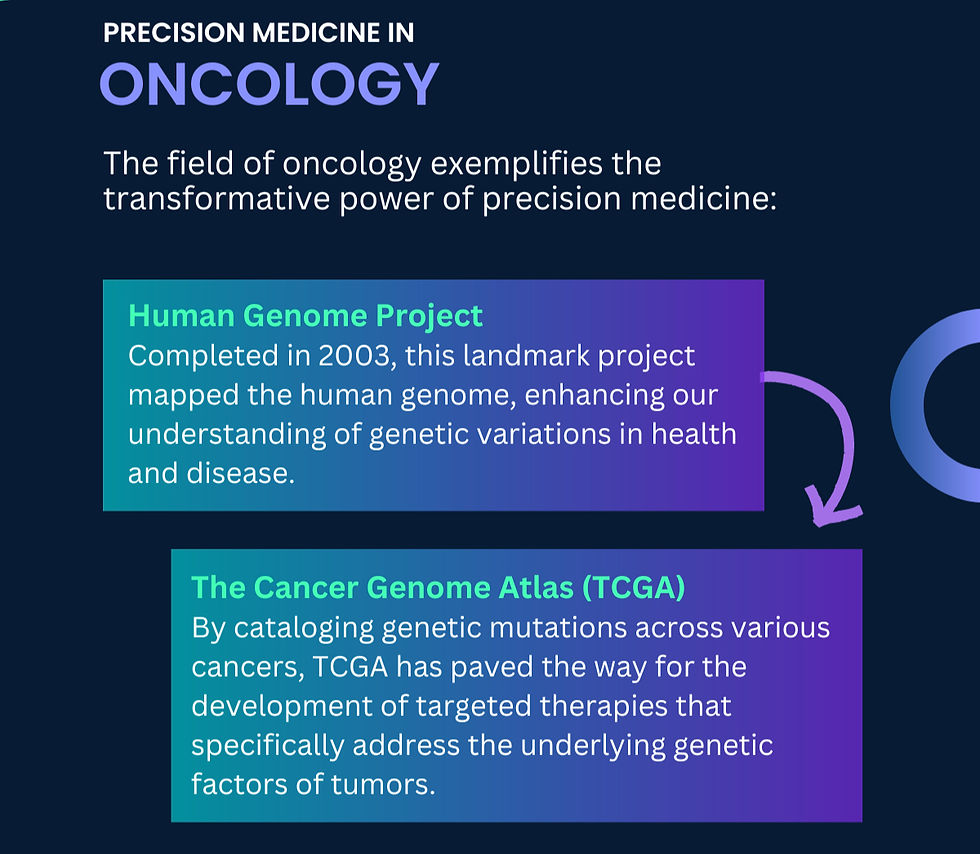
The foundations for precision medicine were laid by the Human Genome Project, which mapped the human genome in 2003 which provided a deeper understanding of how genetic variations contribute to health and disease (Collins et al., 2003). This knowledge is the basis for initiatives like the TCGA, which has been instrumental in cataloging genetic mutations in various cancers, leading to the development of highly targeted cancer treatments.
These advancements in genomics have now begun to extend into other areas of medicine, such as pharmacogenomics, where a patient's genetic makeup can guide drug prescription choices (The Cancer Genome Atlas Research Network, 2013). This acceleration has been bolstered by initiatives like the Precision Medicine Initiative (PMI), launched by President Obama in 2015, and the 21st Century Cures Act of 2016, which streamlined regulatory pathways and increased funding for biomedical research and the approval of personalized treatments (National Institutes of Health [NIH], 2015; 21st Century Cures Act, 2016).
Widespread adoption is still emerging. Significant strides are being made in relating patient data to specific outcomes and in expanding the use of established techniques to addressing certain chronic conditions and rare diseases. Challenges remain in cost, accessibility, and data integration, and more research is needed to develop our understanding of how our individual makeup impacts prevention, treatment, and outcomes.
Moreover, personalization of healthcare requires a change in practices and expectations among both patients and doctors. One initiative, the All of Us Research Program, a cornerstone of the Precision Medicine Initiative, aims to collect data from over one million individuals in the U.S. to better understand how genetics, environment, and lifestyle contribute to health. This large-scale study will help build a vast resource of data that can be used to develop more personalized prevention and treatment strategies, especially for historically underrepresented communities (NIH, 2021).
AI is Critical to Analyzing Big Data
AI drives precision medicine by processing vast amounts of genetic, historical, and lifestyle data, much more than is possible for researchers and doctors to process for each individual patient. Mark Hanson, PhD., says
"AI has a tremendous ability to sort through lots and lots of data, whether it's clinical records, claims records, or whatnot. And then identify patients who might be really good candidates to receive better care in a specialty setting"
(Biomedicial Frontiers, 2024).
However, creating algorithms that are predictive and personalized requires big data. A recommended framework is the 5Vs of data: volume, velocity, variety, veracity, and value (Johnson, et al, 2020). This is a challenge for developers, but is rapidly being overcome.
Howard Rosen, CEO of Nova Insights writes:
“The most promising application of AI technology in healthcare is in the realm of personalized medicine. By leveraging AI algorithms to analyze vast amounts of patient data, healthcare providers can tailor treatments to individual patients based on their unique genetic makeup, medical history, and lifestyle factors.
At the core of personalized medicine is the use of predictive analytics and machine-learning algorithms. These technologies enable healthcare providers to:
Identify at-risk patients: By analyzing patient data, AI can predict which individuals are more likely to develop certain diseases or experience adverse drug reactions.
Optimize treatment plans: AI can help determine the most effective treatment options for individual patients based on their specific characteristics.
Monitor patient outcomes: AI can track patient progress and suggest adjustments to treatment plans as needed to ensure optimal outcomes.
The application of AI in personalized medicine has the potential to revolutionize healthcare by:
Reducing healthcare costs: By identifying at-risk patients early and optimizing treatment plans, AI can help to reduce unnecessary healthcare spending.
Improving patient outcomes: Tailored treatments can lead to better health outcomes, reduced hospitalization rates, and improved quality of life for patients.
Accelerating drug discovery: AI can be used to identify new drug targets and accelerate the development of personalized therapies.
The competitive advantage of AI in personalized medicine lies in its ability to deliver precision and efficiency. By analyzing massive datasets and identifying patterns that humans may miss, AI can provide more accurate and personalized care. This can lead to improved patient outcomes, reduced healthcare costs, and a more efficient healthcare system overall.” Howard Rosen, CEO, Nova Insights
Does Machine Learning Improve Diagnostic Accuracy?
To-date the results in using AI to replace traditional diagnosis techniques have been mixed. A recent study from the NIH highlights both the promise and limitations of AI in this area. While AI models outperformed physicians in closed-book tests by correctly identifying diagnoses, physicians using external reference tools performed better on more difficult cases (NIH, 2024).
Interestingly, the study revealed that while AI could frequently identify the right diagnosis, it often struggled with explaining its reasoning. This gap in interpretability presents a potential risk in clinical settings, as physicians rely on the transparency of diagnostic tools to make informed decisions. The AI model made errors in describing medical images and rationalizing its decisions, even when it arrived at the correct conclusion. This underscores the need for AI tools to complement, rather than replace, human judgment in medical decision-making, particularly in cases requiring nuanced explanations.
In our interviews with entrepreneurs who are applying AI technologies to medical diagnosis we discovered that some new tools are not replacing traditional methods directly but are actually developing entirely new diagnostic methods. For example FibriCheck, which monitors atrial fibrillation using smartphone-based photoplethysmography (PPG), demonstrates this type of innovation.
A clinical study completed in November 2023 validated FibriCheck's ability to monitor atrial fibrillation (AF) in real-world, unsupervised conditions. With a 98.3% sensitivity and 99.9% specificity for AF detection, FibriCheck's use of PPG to detect heart rhythm abnormalities marks a new approach, moving beyond traditional electrocardiograms (ECG) in diagnosing heart conditions (Gruwez et al., 2024). This highlights AI's potential to develop entirely new diagnostic pathways.
Our team at StratCraft predicts that this technology trend is far more revolutionary and impactful then one-to-one replacement of human diagnosis with AI driven analysis.
Advancements in AI Driving Personalized Medicine Forward
AI technologies are being rapidly developed so that certain challenges in development costs and time are being rapidly overcome. Examples of continuing advances include better access to quantitative data and de-identified patient records, enhanced hardware and ongoing algorithm improvements, and a growing library of pre-built models and modules. These advances are unlocking new applications of AI in diagnosis, clinical decision making, medical devices, and automations in medical robotics and, in particular, in predictive medicine.
Better Access to Data For Rapid Product Development
Access to healthcare data has improved with initiatives across multiple hospital systems and universities to create data partnerships. For example, the Mayo Clinic Platform_Accelerate program helps AI companies become market-ready by offering access to experts in regulatory, clinical, technology, and business fields. Most valuable is that startups in this program receive AI model validation support and access to de-identified Mayo Clinic patient data in a secure environment.
Another example is SPOKE (Scalable Precision Medicine Open Knowledge Engine): a comprehensive database of interconnected biological and medical data from UC Davis. This resource integrates data from sources like genetics, clinical phenotypes, environment, and lifestyle to enable researchers, clinicians, and startups to explore disease mechanisms, generate hypotheses, and develop more precise diagnoses and treatments. SPOKE currently connects 19 databases, including UCSF’s de-identified patient data, continuously acquiring new information from research, trials, and health records.
Additionally, Generative Adversarial Networks (GANs) are now capable of generating synthetic medical images that can be used to train other AI models, reducing the need for large, real-world datasets and allowing for faster development of AI-driven diagnostic tools.
Hardware and Algorithm Research Reducing Costs
NVIDIA and Intel have introduced new hardware solutions such as Intel's Gaudi 3 accelerator, which offers training speeds up to 1.7 times faster than previous models. These improvements are crucial for handling complex AI workloads in fields like healthcare, where models require substantial computational resources to process large datasets and make real-time decisions (Spherical Insights, 2024). Enhanced GPUs and TPUs have increased memory bandwidth and processing capabilities, allowing AI models to train and perform more efficiently.
Additionally, algorithmic advancements have played a key role in boosting AI performance. Research shows that in areas such as large language models (LLMs) and computer vision, advancements in AI algorithms are contributing as much to performance improvements as hardware innovations. Some studies suggest that these algorithmic improvements have surpassed hardware advancements, making AI systems more efficient with fewer computational resources (MIT FutureTech, 2024). This combination of enhanced hardware and more efficient algorithms is enabling faster, more scalable AI applications across a wide range of market segments.
Open Access and Pre-Trained Models Simplifying Product Development
One significant advancement in AI is the widespread use of pre-trained models. These models, which have been trained on large, diverse datasets, can be fine-tuned on smaller, more specific datasets, reducing the computational resources and time needed for training. This approach is already proving useful in healthcare, natural language processing (NLP), and computer vision applications. Hugging Face, a popular platform for pre-trained models, has seen more than 150,000 pre-trained models uploaded to its repository as of 2024, representing a dramatic increase from just a few thousand models in 2020 (Hugging Face, 2024).
These pre-trained models are critical for small dataset scenarios where collecting massive amounts of domain-specific data is either costly or impractical. Pre-trained models help overcome this hurdle by leveraging general knowledge learned from large datasets and applying it to specialized medical tasks. Moreover, the introduction of smaller, more efficient models has made it possible to deploy pre-trained models in resource-constrained environments, like mobile devices, without sacrificing too much accuracy. The use of these models has increased across industries, with 92% of companies in AI-driven fields now utilizing some form of transfer learning in their workflows (McKinsey, 2024). For innovators, the proliferation of AI technologies opens up opportunity to build on pre-existing models and data.
Doctors' and Patients' Adoption of New Technologies
While early adopters in the pharmaceutical and specialty care industries are leveraging AI tools already, the wider adoption among doctors and patients remains a challenge. Many healthcare professionals are hesitant to embrace these technologies due to concerns about interpretability, training, and workflow disruption. Doctors need to understand how AI-derived insights work, requiring solutions that are explainable and easy to integrate into their current practice. Similarly, patients need to trust these technologies, which requires clear communication about how AI contributes to their diagnosis and treatment plans. Trust and understanding are essential for overcoming these barriers and accelerating adoption.
Pain Points in Integrating AI into the Healthcare System
Integrating AI into healthcare comes with significant challenges. First, healthcare providers are often slow to adopt new technologies due to deeply ingrained workflows and regulatory hurdles. AI systems need to be integrated into electronic health records (EHRs), clinical decision-making, and patient communication processes. Additionally, many AI solutions face interoperability issues, as healthcare data is often fragmented and stored in different formats. Regulatory challenges further complicate matters, as AI-enabled systems evolve rapidly, while regulatory frameworks struggle to keep pace with the innovation cycles.

Cost Constraints in Commercializing Precision Medicine
The commercialization of AI-driven precision medicine is hindered by cost constraints. While AI has the potential to streamline clinical trials and improve diagnostic accuracy, the upfront costs of developing, implementing, and maintaining these technologies are substantial. For example, developing AI models requires significant computational resources, and integrating these models into healthcare systems demands investment in infrastructure and training. Furthermore, reimbursement policies often lag behind technological advancements, making it difficult for healthcare providers to recoup the costs of adopting AI solutions. This issue is particularly evident in smaller practices and hospitals, where resources are more limited.
Pharmaceutical Industry: The First to Adopt AIs
Pharmaceutical companies are at the forefront of AI adoption, driven by the high cost of drug development and the need to reduce clinical trial timelines and risks. By using AI-powered digital twins and machine learning models, pharmaceutical companies can simulate clinical trials and predict which trial designs are most likely to succeed. This has the potential to save millions of dollars in trial costs and increase the speed of drug approvals. In our interviews with founders, we discovered that biopharmaceutical and therapeutic companies are the "innovators" on the adoption curve, leveraging AI to minimize risks and improve trial efficiency.
Crossing the Chasm to Widespread Adoption
Despite the promise of AI in precision medicine, the healthcare industry is still in the early stages of adoption. As noted in Geoffrey Moore’s Crossing the Chasm model, innovators and early adopters are driving the initial momentum, but for AI technologies to reach mainstream healthcare providers, key challenges must be addressed. Reimbursement models need to evolve, incentives for adopting AI must increase, and AI systems must be designed to explain their conclusions clearly to doctors and nurses. Additionally, regulatory agencies must adapt to the rapid iteration cycles that AI enables. Only by addressing these hurdles can the industry "cross the chasm" and bring AI-enabled precision medicine to traditional hospitals and care providers.
Case Study: Cellular Vehicles – Automating the Last Mile of Cell Therapy to Enable Precision Medicine
Cellular Vehicles is automating the critical last steps of cell therapy delivery. Cell therapies are highly personalized treatments, created from a patient’s own cells or customized to their genetic profile. Today, the processing of these therapies in hospitals is a very manual process: thawing, preparing, and administering cell therapy is only done at large centers with labs and lab technician staff. Its also a manual approach with risks of variability that can negatively impact treatment outcomes.
Cellular Vehicles has developed AI-driven medical robots that automate the final steps in cell therapy delivery, ensuring the consistency and quality control (QC) needed for effective treatment. Their system uses machine learning to assess the quality of the cell therapy product right before it is injected into the patient, a key factor for determining the therapy’s effectiveness. This ensures that each therapy is administered in its optimal state, aligning with the core principles of precision medicine—tailoring treatments to the individual while minimizing variability in care. Cellular Vehicles makes offering cell therapy much cheaper and less cumbersome, making it into a treatment that can be provided in smaller hospital systems and centers.
The AI-powered QC step analyzes cell imagery to detect subtle changes in cell quality, offering biopharmaceutical companies greater insight into the reasons why a treatment may fail or succeed. This data-driven approach helps biopharma companies maintain consistency across hospitals and provides real-time feedback on the quality of the therapies being administered. For healthcare providers, the technology reduces the need to hire and train specialized personnel for cell therapy preparation and guarantees that each therapy is prepared and delivered correctly, enhancing the precision of patient care.
Cellular Vehicles is advancing precision medicine by ensuring that cell therapies—already highly personalized—are delivered with the consistency and quality required to maximize their efficacy.
Future Applications of AI in Healthcare
Investment and market analysis all show that AI is the fastest growing segment of the healthcare industry. A July 2024 Silicon Valley Bank reports "Since 2022, seed and series A pre-money valuations for healthcare companies leveraging AI have outpaced those not utilizing it." Already in 2024 $2.8 billion has already been invested in AI Healthcare with SVB projecting a total of $11.1 billion in VC capital. In our interviews with entrepreneurs we asked each to describe their vision for the impact that is possible with ML technologies in the healthcare industry. A common theme was to see more use cases for AI supporting clinical decision making, driving cost down while increasing access in rural areas, and early detection of diseases leading to better health outcomes.
Clinical Decision Making
One of the key players in AI-enabled clinical decision-making is Google’s DeepMind, which has made significant strides in using AI to assist healthcare providers. DeepMind’s AI models are designed to analyze patient data, including imaging and electronic health records, to provide clinicians with critical insights that can help with diagnosis and treatment planning. For example, DeepMind’s work with Moorfields Eye Hospital in London has led to an AI system capable of diagnosing over 50 eye diseases with accuracy comparable to that of human specialists, demonstrating how AI can complement human expertise in complex medical scenarios.
Integrating AI into clinical decision-making presents a hurdle: Patient and provider trust. Clinicians are hesitant to rely on AI-driven recommendations if they do not fully understand how those recommendations are made, a concern often referred to as the “black box” problem. AI systems may produce accurate results but the decision-making process must be made transparent and comprehensible to the doctor .
In one interview, the founder shared:
"Doctors make different mistakes than AI but the mistakes that AI makes are obvious to doctors."
Even when the black box problem is solved the differences in how a computer vs a human processes information are still evident. Doctors offices have to develop policies and guidelines for how conflicts between AI’s suggestions and clinician’s judgment would be handled once we're relying on these technologies for driving decisions about treatment. To achieve the advances that are possible we need to deploy these systems to prove themselves in real-world clinical settings but the technological advances will likely outpace the technology adoption by years, if not decades.

Improving Access and Reducing Cost
Artificial intelligence solutions provide hope that some expenses, inefficiencies, and costly mistakes in healthcare could become obsolete. More doctors, even those in smaller practices or rural areas, could have access cutting-edge tools, most recent knowledge, and diagnostic support that were previously limited to large, specialized institutions. By reducing the time and personnel required for advanced diagnosis techniques, AI-enabled devices can lower barriers to personalized treatments, ensuring that high-quality care is available to more patients.
Many people are predicting that the AI revolution will lead to long term cost reduction but to-date the results are mixed. In a recent study of new technology adoption in dental health the study found that "the use of AI was associated with a modest improvement in outcomes. All benefits were highly dependent on treatment effects assumed after diagnosis and were very sensitive to the fee paid for the use of AI." (Rossi, et.al. 2022)
Authors:
About the Applications of AI in Healthcare Research Event:
Goals of the Research Project
AI technology is rapidly transforming the healthcare and life-sciences industry. The StratCraft team is researching the state of adoption of AI in healthcare and the challenges and opportunities faced by entrepreneurs developing these solutions. Our goal is to provide valuable knowledge to investors, entrepreneurs and their commercialization partners, and the decision makers who are at the forefront of testing, purchasing, and using these new technologies.
The research results will be published on the StratCraft website in a white paper and a dynamic database including quotes from all the experts, key opinion leaders, entrepreneurs, and investors interviewed.
Methods of Research
StratCraft Applications of AI in Healthcare Research Event draws on in-person interviews conducted by our team and deep research on frameworks and concepts for how AI development and adoption is progressing. Our project covers important developments in regulation and policy, investment and financing, customer expectations and behaviors, technology adoption and clinical outcomes, and the competitive landscape and unique advantages of the unique technologies being worked on by both startups and established data analytics, NLP, and AI companies.
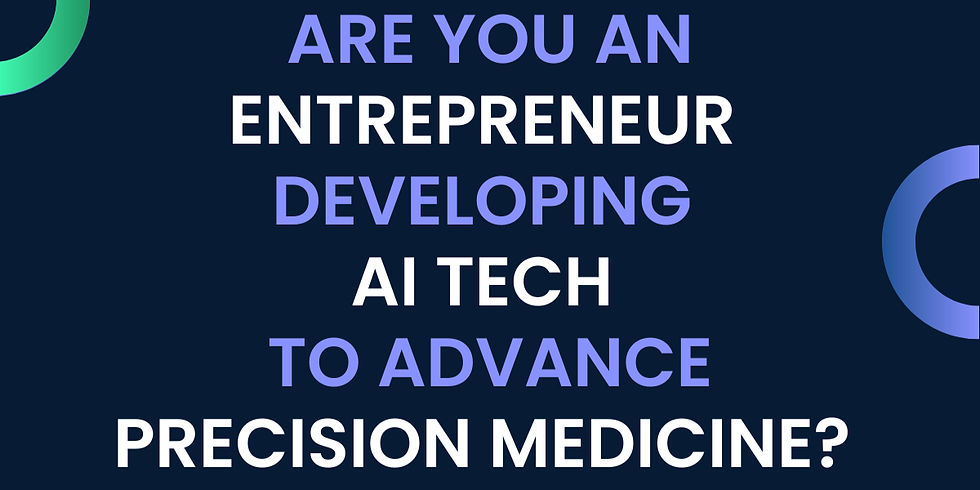
Connect with us to be interviewed for our upcoming White Paper on Applications of AI in Healthcare
StratCraft Partners are experts in commercializing emerging technologies that benefit human life. We work with organizations that have portfolios of cutting edge technologies to help them develop comprehensive commercialization strategy that ensures the success of multiple projects. We also work with deep tech ventures and deep tech incubators to support growth and scaling of emerging technologies that save human life.
Sources
UC Davis Health Center for Precision Medicine and Data Sciences. (n.d.). What is Precision Medicine? Retrieved September 16, 2024, from https://health.ucdavis.edu/precision-medicine/what-is-precision-medicine.html
Hood, L., & Price, N. (2023). The Age of Scientific Wellness: Why the Future of Medicine Is Personalized, Predictive, Data-Rich, and in Your Hands. Harvard University Press. https://doi.org/10.2307/jj.362389
Collins, F. S., Morgan, M., & Patrinos, A. (2003). The Human Genome Project: Lessons from large-scale biology. Science, 300(5617), 286-290. https://doi.org/10.1126/science.1084564
National Institutes of Health. (2015). Precision Medicine Initiative: Overview. NIH. https://www.nih.gov/precision-medicine-initiative
The Cancer Genome Atlas Research Network. (2013). The Cancer Genome Atlas Pan-Cancer analysis project. Nature Genetics, 45(10), 1113–1120. https://doi.org/10.1038/ng.2764
National Institutes of Health. (2021). All of Us Research Program overview. NIH. https://allofus.nih.gov/about
Tyshlek, D. (Host). (2024, June 26). Dr. Mark Hanson on the Evolution of Healthcare Delivery, AI in Heart Health and Wireless Medical Devices (No. 6) [Audio Podcast Episode]. In Biomedical Frontiers: Stories with Innovators in Healthcare. https://rss.com/podcasts/biomedicalfrontiers/1540595/
National Institutes of Health. (2024). NIH findings shed light on risks and benefits of integrating AI into medical decision-making. NIH News Releases. Retrieved from https://www.nih.gov/news-events/news-releases/nih-findings-shed-light-risks-benefits-integrating-ai-into-medical-decision-making.
Gruwez, H., Ezzat, D., Van Puyvelde, T., et al. (2024). Real-world validation of smartphone-based photoplethysmography for rate and rhythm monitoring in atrial fibrillation. Europace, 26, euae065. https://doi.org/10.1093/europace/euae065
Mayo Clinic Platform_Accelerate program begins with four AI startups. (2022, March 23). [Press release]. Retrieved September 17, 2024, from https://newsnetwork.mayoclinic.org/discussion/3-23-mayo-clinic-platform_accelerate-program-begins-with-four-ai-startups/
Spherical Insights. (2024). Top 6 AI trends for 2024: Key stats, growth projections, and insights. Spherical Insights. https://www.sphericalinsights.com
MIT FutureTech. (2024). What drives progress in AI? Trends in algorithms. MIT FutureTech. https://futuretech.mit.edu
Hugging Face. (2024). Hugging Face Model Hub Statistics. Hugging Face. https://huggingface.co/models
McKinsey. (2024). The state of AI in early 2024. McKinsey & Company. https://www.mckinsey.com
Moore, G. A. (1991). Crossing the chasm: marketing and selling disruptive products to mainstream customers. HarperCollins
Bank, S. V. B. a. D. O. F. C. (2024, June 11). AI investments dominate healthcare; Silicon Valley Bank releases new AI Patient Journey report. Silicon Valley Bank. https://www.svb.com/news/company-news/ai-investments-dominate-healthcare--silicon-valley-bank-releases-new-ai-patient-journey-report/#:~:text=The%20report%20found%20that%20%242.8,it%20has%20been%20since%202021.
Moore, T. J., Heyward, J., Anderson, G., & Alexander, G. C. (2020a). Variation in the estimated costs of pivotal clinical benefit trials supporting the US approval of New Therapeutic Agents, 2015–2017: A cross-sectional study. BMJ Open, 10(6). https://doi.org/10.1136/bmjopen-2020-038863
Gomez Rossi, J., Rojas-Perilla, N., Krois, J., & Schwendicke, F. (2022). Cost-effectiveness of Artificial Intelligence as a Decision-Support System Applied to the Detection and Grading of Melanoma, Dental Caries, and Diabetic Retinopathy. JAMA network open, 5(3), e220269. https://doi.org/10.1001/jamanetworkopen.2022.0269
Commenti